1 Step Adams Moulton#
John S Butler#
john.s.butler@tudublin.ie
Course Notes Github
This notebook implements the 1 step Adams Moulton method for three different population intial value problems.
Formula#
The general 1 step Adams-Moulton method for the first order differential equation
numerical approximates \(y\) the at time point \(t_i\) as \(w_i\) with the formula:
for \(i=0,...,N-1\), where
and \(h\) is the stepsize.
To illustrate the method we will apply it to three intial value problems:
1. Linear#
Consider the linear population Differential Equation
with the initial condition,
2. Non-Linear Population Equation#
Consider the non-linear population Differential Equation
with the initial condition,
3. Non-Linear Population Equation with an oscillation#
Consider the non-linear population Differential Equation with an oscillation
with the initial condition,
Setting up Libraries#
## Library
import numpy as np
import math
import pandas as pd
%matplotlib inline
import matplotlib.pyplot as plt # side-stepping mpl backend
import matplotlib.gridspec as gridspec # subplots
import warnings
warnings.filterwarnings("ignore")
Discrete Interval#
The continuous time \(a\leq t \leq b \) is discretised into \(N\) points seperated by a constant stepsize
Here the interval is \(2000\leq t \leq 2020,\)
This gives the 201 discrete points:
This is generalised to
The plot below shows the discrete time steps:
### DISCRETE TIME
N=20
t_end=2020.0
t_start=2000.0
h=((t_end-t_start)/N)
t=np.arange(t_start,t_end+h/2,h)
## PLOTS TIME
fig = plt.figure(figsize=(10,4))
plt.plot(t,0*t,'o:',color='red')
plt.title('Illustration of discrete time points for h=%s'%(h))
plt.show()
len(t)
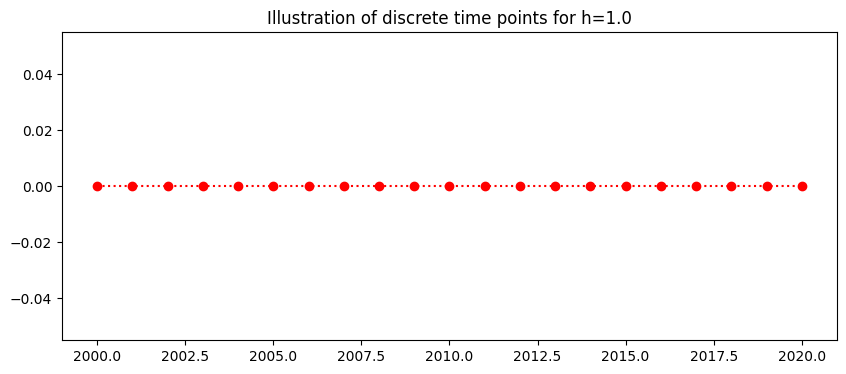
21
1. Linear Population Equation#
Exact Solution#
The linear population equation
with the initial condition,
has a known exact (analytic) solution
Specific 1 step Adams Moulton#
The specific 1 step Adams Moulton for the linear population equation is:
where
## THIS IS THE RIGHT HANDSIDE OF THE LINEAR POPULATION DIFFERENTIAL
## EQUATION
def linfun(t,w):
ftw=0.1*w
return ftw
re-arranging,
### INSERT METHOD HERE
w=np.zeros(N+1) # a list of 2000+1 zeros
w[0]=6 # INITIAL CONDITION
for i in range(0,N):
w[i+1]=(w[i]+h/2*(linfun(t[i],w[i])))/(1-0.1*h/2)
Plotting Results#
## PLOTTING METHOD
y=6*np.exp(0.1*(t-2000)) # EXACT SOLUTION
fig = plt.figure(figsize=(8,4))
plt.plot(t,w,'o:',color='purple',label='Taylor')
plt.plot(t,y,'s:',color='black',label='Exact')
plt.legend(loc='best')
plt.show()
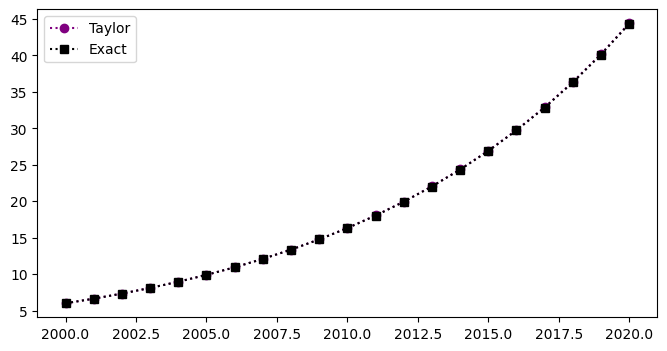
Table#
The table below shows the time, the numerical approximation, \(w\), the exact solution, \(y\), and the exact error \(|y(t_i)-w_i|\) for the linear population equation:
d = {'time t_i': t, 'Adams Approx w': w}
df = pd.DataFrame(data=d)
df
time t_i | Adams Approx w | |
---|---|---|
0 | 2000.0 | 6.000000 |
1 | 2001.0 | 6.631579 |
2 | 2002.0 | 7.329640 |
3 | 2003.0 | 8.101181 |
4 | 2004.0 | 8.953937 |
5 | 2005.0 | 9.896456 |
6 | 2006.0 | 10.938189 |
7 | 2007.0 | 12.089577 |
8 | 2008.0 | 13.362164 |
9 | 2009.0 | 14.768708 |
10 | 2010.0 | 16.323308 |
11 | 2011.0 | 18.041551 |
12 | 2012.0 | 19.940662 |
13 | 2013.0 | 22.039679 |
14 | 2014.0 | 24.359645 |
15 | 2015.0 | 26.923819 |
16 | 2016.0 | 29.757905 |
17 | 2017.0 | 32.890316 |
18 | 2018.0 | 36.352454 |
19 | 2019.0 | 40.179029 |
20 | 2020.0 | 44.408400 |
2. Non-Linear Population Equation#
with the initial condition,
Specific 1 step Adams-Moutlon method for the Non-Linear Population Equation#
The specific Adams-Moulton difference equation for the non-linear population equations is:
re-arranging
for \(i=0,...,199\), where \(w_i\) is the numerical approximation of \(y\) at time \(t_i\), with step size \(h\) and the initial condition
PROBLEM WE CANNOT MOVE THE SQUARED (NON-LINEAR TERM) TO THE RIGHT HAND SIDE SO WE CAN SOLVE FOR w[i+1]. For this reason we will use a predictor-corrector method, The predictor will be the 2-step Adams Bashforth
with the corrector being the 1-step Adams Moulton,
for i=1,…200.
def nonlinfun(t,w):
ftw=0.2*w-0.01*w*w
return ftw
### INSERT METHOD HERE
w=np.zeros(N+1)
w_p=np.zeros(N+1)
w[0]=6
w[1]=6.084 # FROM THE THE TAYLOR METHOD
w_p[0]=6
w_p[1]=6.084 # FROM THE THE TAYLOR METHOD
for n in range(1,N):
## Predictor
w_p[n+1]=w[n]+h/2*(3*nonlinfun(t[n],w[n])-
nonlinfun(t[n-1],w[n-1]))
## Corrector
w[n+1]=w[n]+h/2*(nonlinfun(t[n+1],w_p[n+1])+
nonlinfun(t[n],w[n]))
Results#
The plot below shows the numerical approximation, \(w\) (circles) for the non-linear population equation:
fig = plt.figure(figsize=(8,4))
plt.plot(t,w,'-.',color='purple',label='Correcter ')
plt.plot(t,w_p,':',color='red',label='Predictor ')
plt.title('Non Linear Population Equation')
plt.legend(loc='best')
plt.xlabel('time (yrs)')
plt.ylabel('Population in billions')
plt.show()
fig = plt.figure(figsize=(8,4))
plt.plot(t,np.abs(w-w_p),':',color='purple',label='|w-w_p| ')
plt.title('Comparison of Predictor and Corrector')
plt.legend(loc='best')
plt.xlabel('time (yrs)')
plt.ylabel('Population in billions')
plt.show()
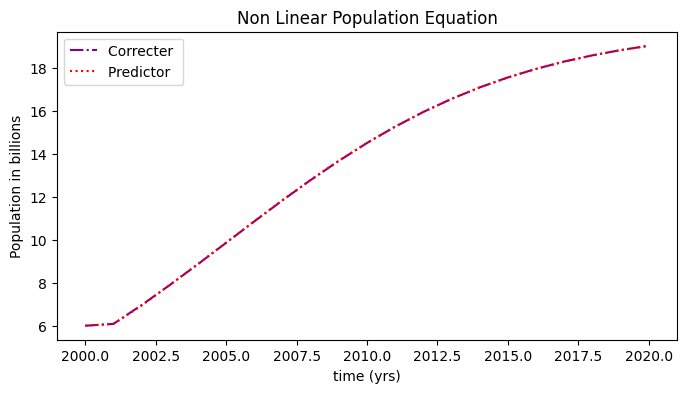
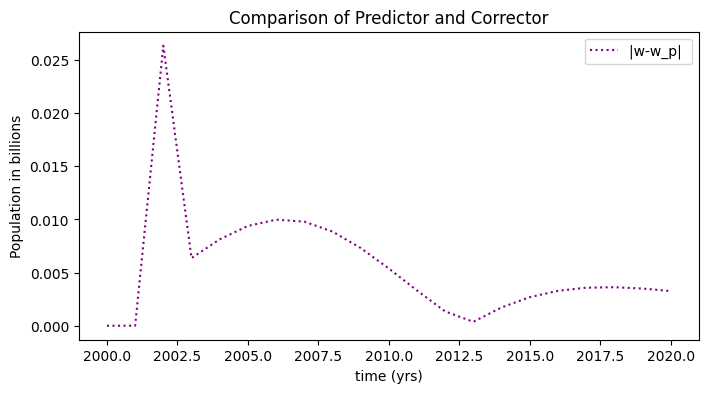
Table#
The table below shows the time and the numerical approximation, \(w\), for the non-linear population equation:
d = {'time t_i': t, 'Adams Approx w': w}
df = pd.DataFrame(data=d)
df
time t_i | Adams Approx w | |
---|---|---|
0 | 2000.0 | 6.000000 |
1 | 2001.0 | 6.084000 |
2 | 2002.0 | 6.960322 |
3 | 2003.0 | 7.892040 |
4 | 2004.0 | 8.863456 |
5 | 2005.0 | 9.856908 |
6 | 2006.0 | 10.853082 |
7 | 2007.0 | 11.832473 |
8 | 2008.0 | 12.776881 |
9 | 2009.0 | 13.670688 |
10 | 2010.0 | 14.501747 |
11 | 2011.0 | 15.261810 |
12 | 2012.0 | 15.946493 |
13 | 2013.0 | 16.554881 |
14 | 2014.0 | 17.088907 |
15 | 2015.0 | 17.552635 |
16 | 2016.0 | 17.951549 |
17 | 2017.0 | 18.291930 |
18 | 2018.0 | 18.580348 |
19 | 2019.0 | 18.823293 |
20 | 2020.0 | 19.026909 |
3. Non-Linear Population Equation with an oscilation#
with the initial condition,
Specific 2 Step Adams Moulton for the Non-Linear Population Equation with an oscilation#
The specific Adams-Moulton difference equation for the non-linear population equations is:
for \(i=1,...,199\), where \(w_i\) is the numerical approximation of \(y\) at time \(t_i\), with step size \(h\) and the initial condition
As \(w_1\) is required for the method but unknown we will use the numerical solution of a one step method to approximate the value. Here, we use the 2nd order Runge Kutta approximation (see Runge Kutta notebook )
PROBLEM WE CANNOT MOVE THE SQUARED (NON-LINEAR TERM) TO THE RIGHT HAND SIDE SO WE CAN SOLVE FOR w[i+1]. For this reason we will use a predictor-corrector method, The predictor will be the 2-step Adams Bashforth
with the corrector being the 1-step Adams Moulton,
def nonlin_oscfun(t,w):
ftw=0.2*w-0.01*w*w+np.sin(2*np.math.pi*t)
return ftw
## INSERT METHOD HERE
w=np.zeros(N+1)
w[0]=6
w[1]=6.11
for n in range(1,N):
w[n+1]=w[n]+h/2
Results#
The plot below shows the numerical approximation, \(w\) (circles) for the non-linear population equation:
fig = plt.figure(figsize=(8,4))
plt.plot(t,w,'o:',color='purple',label='Adams-Moulton')
plt.title('Population Equation with seasonal oscilation')
plt.xlabel('time (yrs)')
plt.ylabel('Population in Billions')
plt.legend(loc='best')
plt.show()
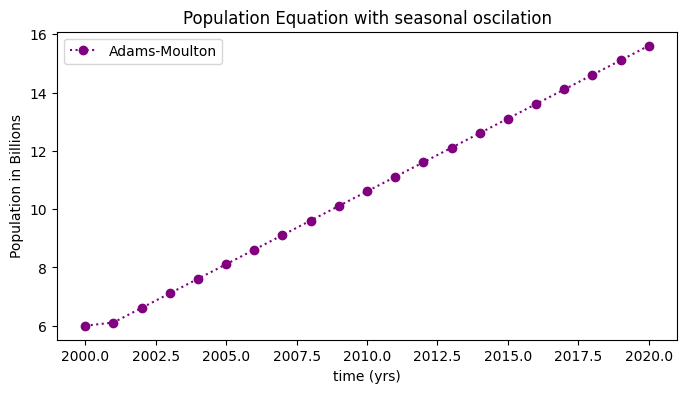
Table#
The table below shows the time and the numerical approximation, \(w\), for the non-linear population equation:
d = {'time t_i': t, 'Adams Approx w': w}
df = pd.DataFrame(data=d)
df
time t_i | Adams Approx w | |
---|---|---|
0 | 2000.0 | 6.00 |
1 | 2001.0 | 6.11 |
2 | 2002.0 | 6.61 |
3 | 2003.0 | 7.11 |
4 | 2004.0 | 7.61 |
5 | 2005.0 | 8.11 |
6 | 2006.0 | 8.61 |
7 | 2007.0 | 9.11 |
8 | 2008.0 | 9.61 |
9 | 2009.0 | 10.11 |
10 | 2010.0 | 10.61 |
11 | 2011.0 | 11.11 |
12 | 2012.0 | 11.61 |
13 | 2013.0 | 12.11 |
14 | 2014.0 | 12.61 |
15 | 2015.0 | 13.11 |
16 | 2016.0 | 13.61 |
17 | 2017.0 | 14.11 |
18 | 2018.0 | 14.61 |
19 | 2019.0 | 15.11 |
20 | 2020.0 | 15.61 |