2 Step Adam Bashforth#
John S Butler#
john.s.butler@tudublin.ie
Course Notes Github
This notebook implements the 2 step Adams Bashforth method for three different population intial value problems.
Formula#
The general 2 step Adams-Bashforth method for the first order differential equation
numerical approximates \(y\) the at time point \(t_i\) as \(w_i\) with the formula:
for \(i=0,...,N-1\), where
and \(h\) is the stepsize.
To illustrate the method we will apply it to three intial value problems:
1. Linear#
Consider the linear population Differential Equation
with the initial condition,
2. Non-Linear Population Equation#
Consider the non-linear population Differential Equation
with the initial condition,
3. Non-Linear Population Equation with an oscillation#
Consider the non-linear population Differential Equation with an oscillation
with the initial condition,
Setting up Libraries#
## Library
import numpy as np
import math
import pandas as pd
%matplotlib inline
import matplotlib.pyplot as plt # side-stepping mpl backend
import matplotlib.gridspec as gridspec # subplots
import warnings
warnings.filterwarnings("ignore")
Discrete Interval#
The continuous time \(a\leq t \leq b \) is discretised into \(N\) points seperated by a constant stepsize
Here the interval is \(2000\leq t \leq 2020,\)
This gives the 201 discrete points:
This is generalised to
The plot below shows the discrete time steps:
### DISCRETE TIME
N=20
t_end=2020.0
t_start=2000.0
h=((t_end-t_start)/N)
t=np.arange(t_start,t_end+h/2,h)
## PLOTS TIME
fig = plt.figure(figsize=(10,4))
plt.plot(t,0*t,'o:',color='red')
plt.title('Illustration of discrete time points for h=%s'%(h))
plt.show()
len(t)
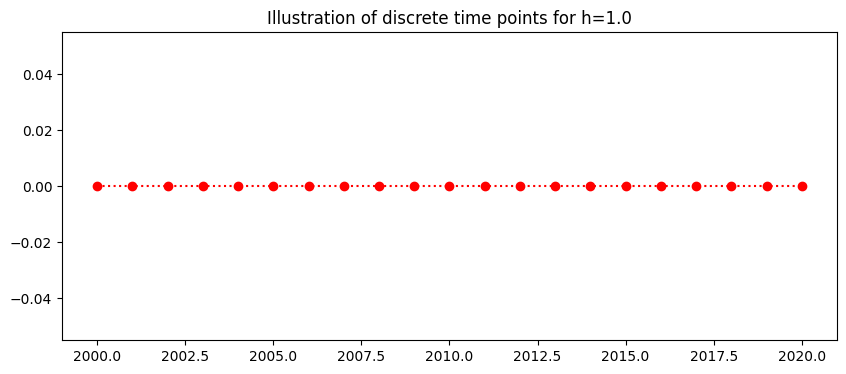
21
1. Linear Population Equation#
Exact Solution#
The linear population equation
with the initial condition,
has a known exact (analytic) solution
Specific 2 step Adams Bashforth#
The specific 2 step Adams Bashforth for the linear population equation is:
where
## THIS IS THE RIGHT HANDSIDE OF THE LINEAR POPULATION DIFFERENTIAL
## EQUATION
def linfun(t,w):
ftw=0.1*w
return ftw
this gives
for \(i=1,...,199\), where \(w_i\) is the numerical approximation of \(y\) at time \(t_i\), with step size \(h\) and the initial condition
### INSERT METHOD HERE
w=np.zeros(N+1) # a list of 2000+1 zeros
w[0]=6 # INITIAL CONDITION
w[1]=6.06
for i in range(1,N):
w[i+1]=w[i]+h/2*(3*linfun(t[i],w[i])-linfun(t[i-1],w[i-1]))
Plotting Results#
## PLOTTING METHOD
y=6*np.exp(0.1*(t-2000)) # EXACT SOLUTION
fig = plt.figure(figsize=(8,4))
plt.plot(t,w,'o:',color='purple',label='Taylor')
plt.plot(t,y,'s:',color='black',label='Exact')
plt.legend(loc='best')
plt.show()
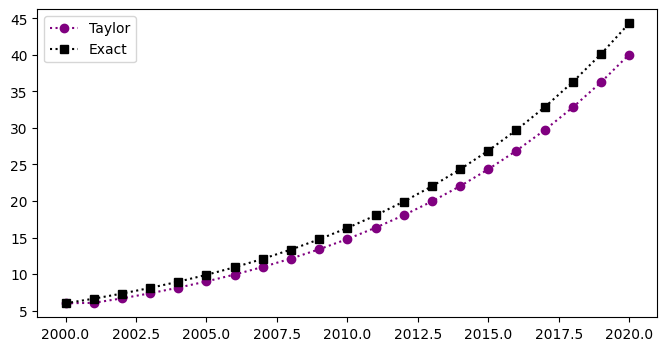
Table#
The table below shows the time, the numerical approximation, \(w\), the exact solution, \(y\), and the exact error \(|y(t_i)-w_i|\) for the linear population equation:
d = {'time t_i': t, 'Adams w': w,'Exact y(t_i)':y,'Exact Error |w_i-y_i|':np.abs(y-w)}
df = pd.DataFrame(data=d)
df
time t_i | Adams w | Exact y(t_i) | Exact Error |w_i-y_i| | |
---|---|---|---|---|
0 | 2000.0 | 6.000000 | 6.000000 | 0.000000 |
1 | 2001.0 | 6.060000 | 6.631026 | 0.571026 |
2 | 2002.0 | 6.669000 | 7.328417 | 0.659417 |
3 | 2003.0 | 7.366350 | 8.099153 | 0.732803 |
4 | 2004.0 | 8.137852 | 8.950948 | 0.813096 |
5 | 2005.0 | 8.990213 | 9.892328 | 0.902115 |
6 | 2006.0 | 9.931852 | 10.932713 | 1.000861 |
7 | 2007.0 | 10.972119 | 12.082516 | 1.110397 |
8 | 2008.0 | 12.121345 | 13.353246 | 1.231901 |
9 | 2009.0 | 13.390940 | 14.757619 | 1.366678 |
10 | 2010.0 | 14.793514 | 16.309691 | 1.516177 |
11 | 2011.0 | 16.342994 | 18.024996 | 1.682002 |
12 | 2012.0 | 18.054768 | 19.920702 | 1.865934 |
13 | 2013.0 | 19.945833 | 22.015780 | 2.069947 |
14 | 2014.0 | 22.034970 | 24.331200 | 2.296230 |
15 | 2015.0 | 24.342924 | 26.890134 | 2.547211 |
16 | 2016.0 | 26.892614 | 29.718195 | 2.825581 |
17 | 2017.0 | 29.709360 | 32.843684 | 3.134325 |
18 | 2018.0 | 32.821133 | 36.297885 | 3.476752 |
19 | 2019.0 | 36.258835 | 40.115367 | 3.856532 |
20 | 2020.0 | 40.056603 | 44.334337 | 4.277733 |
2. Non-Linear Population Equation#
with the initial condition,
Specific 2 step Adams-Bashforth method for the Non-Linear Population Equation#
The specific Adams-Bashforth difference equation for the non-linear population equations is:
for \(i=1,...,199\), where \(w_i\) is the numerical approximation of \(y\) at time \(t_i\), with step size \(h\) and the initial condition
To solve the 2 step method we need a value for \(w_1\), here, we will use the approximation from the 2nd order Taylor method (see other notebook),
def nonlinfun(t,w):
ftw=0.2*w-0.01*w*w
return ftw
### INSERT METHOD HERE
w=np.zeros(N+1)
w[0]=6
w[1]=6.084 # FROM THE THE TAYLOR METHOD
for n in range(1,N):
w[n+1]=w[n]+h/2*(3*nonlinfun(t[n],w[n])-nonlinfun(t[n-1],w[n-1]))
Results#
The plot below shows the numerical approximation, \(w\) (circles) for the non-linear population equation:
fig = plt.figure(figsize=(8,4))
plt.plot(t,w,'o:',color='purple',label='2 step Adams Method ')
plt.title('Non Linear Population Equation')
plt.legend(loc='best')
plt.xlabel('time (yrs)')
plt.ylabel('Population in billions')
plt.show()
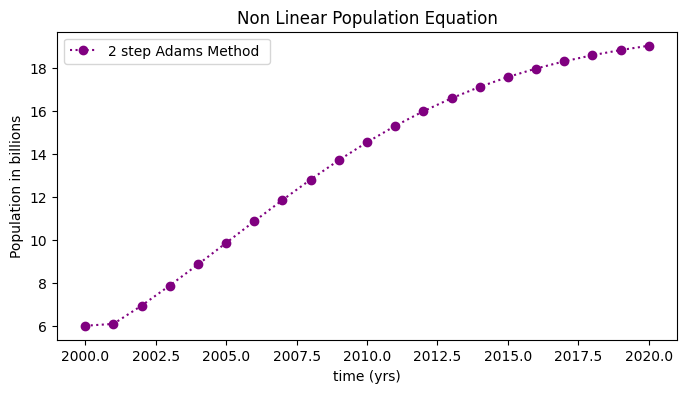
Table#
The table below shows the time and the numerical approximation, \(w\), for the non-linear population equation:
d = {'time t_i': t, 'Adams approx of non-linear w': w}
df = pd.DataFrame(data=d)
df
time t_i | Adams approx of non-linear w | |
---|---|---|
0 | 2000.0 | 6.000000 |
1 | 2001.0 | 6.084000 |
2 | 2002.0 | 6.933974 |
3 | 2003.0 | 7.869642 |
4 | 2004.0 | 8.848568 |
5 | 2005.0 | 9.851373 |
6 | 2006.0 | 10.857671 |
7 | 2007.0 | 11.846747 |
8 | 2008.0 | 12.799268 |
9 | 2009.0 | 13.698782 |
10 | 2010.0 | 14.532747 |
11 | 2011.0 | 15.292965 |
12 | 2012.0 | 15.975461 |
13 | 2013.0 | 16.579947 |
14 | 2014.0 | 17.109042 |
15 | 2015.0 | 17.567443 |
16 | 2016.0 | 17.961143 |
17 | 2017.0 | 18.296777 |
18 | 2018.0 | 18.581128 |
19 | 2019.0 | 18.820774 |
20 | 2020.0 | 19.021862 |
3. Non-Linear Population Equation with an oscilation#
with the initial condition,
Specific 2 Step Adams Bashforth for the Non-Linear Population Equation with an oscilation#
To write the specific
for \(i=1,...,199\), where \(w_i\) is the numerical approximation of \(y\) at time \(t_i\), with step size \(h\) and the initial condition
As \(w_1\) is required for the method but unknown we will use the numerical solution of a one step method to approximate the value. Here, we use the 2nd order Runge Kutta approximation (see Runge Kutta notebook )
def nonlin_oscfun(t,w):
ftw=0.2*w-0.01*w*w+np.sin(2*np.math.pi*t)
return ftw
## INSERT METHOD HERE
w=np.zeros(N+1)
w[0]=6
w[1]=6.11
for n in range(1,N):
w[n+1]=w[n]+h/2*(3*nonlin_oscfun(t[n],w[n])
-nonlin_oscfun(t[n-1],w[n-1]))
Results#
The plot below shows the numerical approximation, \(w\) (circles) for the non-linear population equation:
fig = plt.figure(figsize=(8,4))
plt.plot(t,w,'o:',color='purple',label='Adams-Bashforth')
plt.title('Population Equation with seasonal oscilation')
plt.xlabel('time (yrs)')
plt.ylabel('Population in Billions')
plt.legend(loc='best')
plt.show()
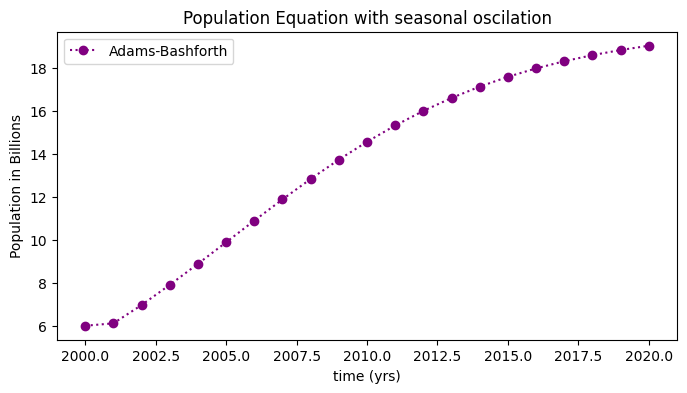
Table#
The table below shows the time and the numerical approximation, \(w\), for the non-linear population equation with oscilations:
d = {'time t_i': t, 'Adams Approx w': w}
df = pd.DataFrame(data=d)
df
time t_i | Adams Approx w | |
---|---|---|
0 | 2000.0 | 6.000000 |
1 | 2001.0 | 6.110000 |
2 | 2002.0 | 6.963018 |
3 | 2003.0 | 7.900330 |
4 | 2004.0 | 8.880317 |
5 | 2005.0 | 9.883555 |
6 | 2006.0 | 10.889620 |
7 | 2007.0 | 11.877816 |
8 | 2008.0 | 12.828881 |
9 | 2009.0 | 13.726473 |
10 | 2010.0 | 14.558187 |
11 | 2011.0 | 15.315964 |
12 | 2012.0 | 15.995957 |
13 | 2013.0 | 16.597982 |
14 | 2014.0 | 17.124739 |
15 | 2015.0 | 17.580977 |
16 | 2016.0 | 17.972719 |
17 | 2017.0 | 18.306611 |
18 | 2018.0 | 18.589435 |
19 | 2019.0 | 18.827758 |
20 | 2020.0 | 19.027711 |